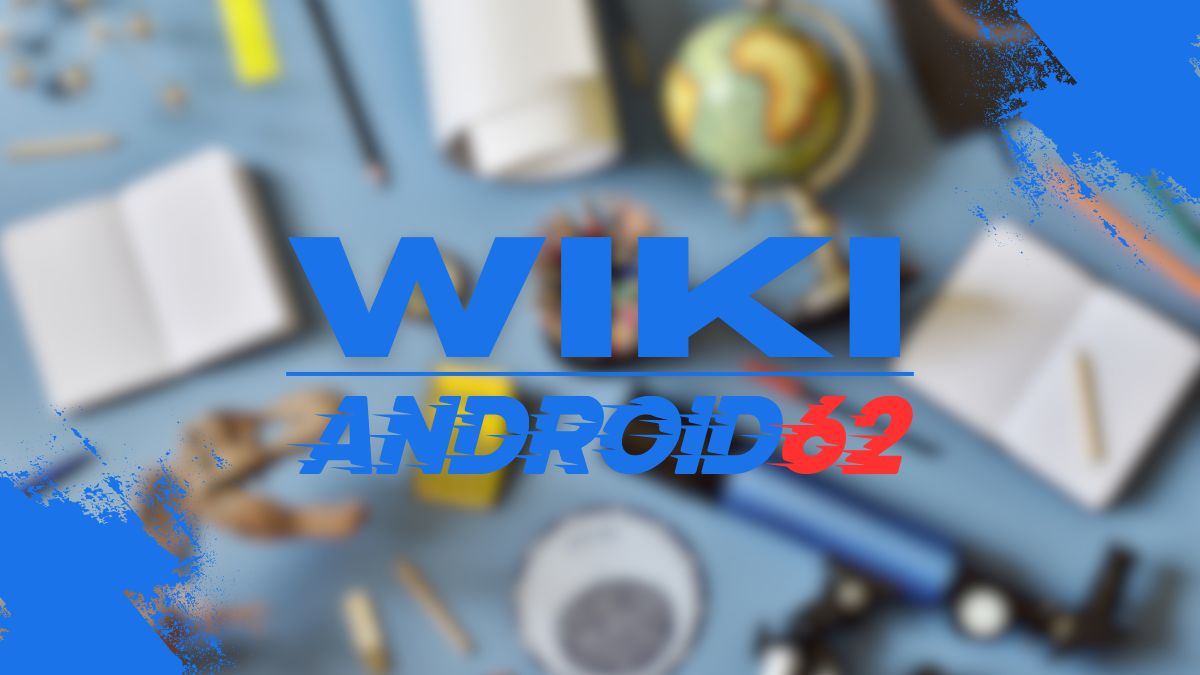
Introduction
Object recognition is a critical function of various systems, including artificial intelligence, robotics, computer vision, and neuroscience. It involves identifying and categorizing objects in the visual field based on their features and characteristics. This process plays a crucial role in enabling machines to interact with the physical world and make intelligent decisions.
Key Points:
- Object recognition is a complex cognitive function that involves the processing of visual information to identify and categorize objects.
- It plays a vital role in various applications such as image and video analysis, autonomous navigation, object tracking, and augmented reality.
- Object recognition is essential for smart technologies to understand and interpret the surrounding environment to perform tasks efficiently.
- Advancements in machine learning and deep learning have significantly improved object recognition capabilities in recent years.
Importance of Object Recognition
Object recognition is crucial for enabling machines and systems to perceive and interpret the visual world accurately. Here are some key reasons why object recognition is a major function:
- Facilitates autonomous navigation: Object recognition is essential for autonomous vehicles and robots to perceive and navigate their surroundings safely and efficiently.
- Enhances security and surveillance: Object recognition technology is used in security systems for detecting and tracking objects of interest, such as intruders or suspicious activities.
- Improves image and video processing: Object recognition algorithms play a crucial role in image and video analysis applications, including content-based image retrieval and video surveillance.
- Enables augmented reality experiences: Object recognition enables augmented reality (AR) applications to overlay digital content onto real-world objects, creating immersive user experiences.
Challenges in Object Recognition
Despite its importance, object recognition poses several challenges due to the complexities of visual data and variability in object appearance. Some of the key challenges in object recognition include:
- Variability in object appearance: Objects can exhibit variations in size, shape, color, texture, and pose, making it challenging to accurately recognize them under different conditions.
- Occlusions and clutter: Objects in the real world may be partially occluded or surrounded by other objects, leading to difficulties in segmenting and recognizing them.
- Limited training data: Building robust object recognition models requires a large and diverse dataset for training, which may not always be readily available.
- Real-time processing requirements: Many applications necessitate real-time object recognition, which poses additional constraints on computational resources and efficiency.
Advancements in Object Recognition
Recent advancements in artificial intelligence and computer vision have revolutionized object recognition capabilities. Here are some key developments that have significantly improved object recognition performance:
- Deep learning: Deep learning techniques, particularly convolutional neural networks (CNNs), have shown remarkable success in object recognition tasks by learning hierarchical features from raw image data.
- Transfer learning: Transfer learning enables models trained on large datasets to be fine-tuned for specific object recognition tasks with limited data, improving performance and generalization.
- Object detection frameworks: Object detection frameworks like YOLO (You Only Look Once) and Faster R-CNN have advanced object recognition capabilities by efficiently detecting and localizing objects in images.
- Interactive and multimodal approaches: Interactive object recognition and multimodal fusion techniques combine visual information with other modalities such as text or audio to improve recognition accuracy.
Future Directions in Object Recognition
The future of object recognition holds promising opportunities for further advancements and applications. Here are some potential directions for the future of object recognition:
- Robustness to adversarial attacks: Developing object recognition models that are resilient to adversarial attacks and can accurately recognize objects in challenging environments.
- Continual learning and adaptation: Implementing systems that can continuously learn and adapt to new objects and environments to enhance long-term performance.
- Combining symbolic and subsymbolic approaches: Integrating symbolic reasoning with deep learning techniques for more explainable and interpretable object recognition systems.
- Scene understanding and context-aware recognition: Enhancing object recognition by considering contextual information and scene understanding to improve recognition accuracy.
- Collaborative object recognition: Enabling collaborative object recognition among multiple agents or devices to enhance scalability and efficiency in complex environments.