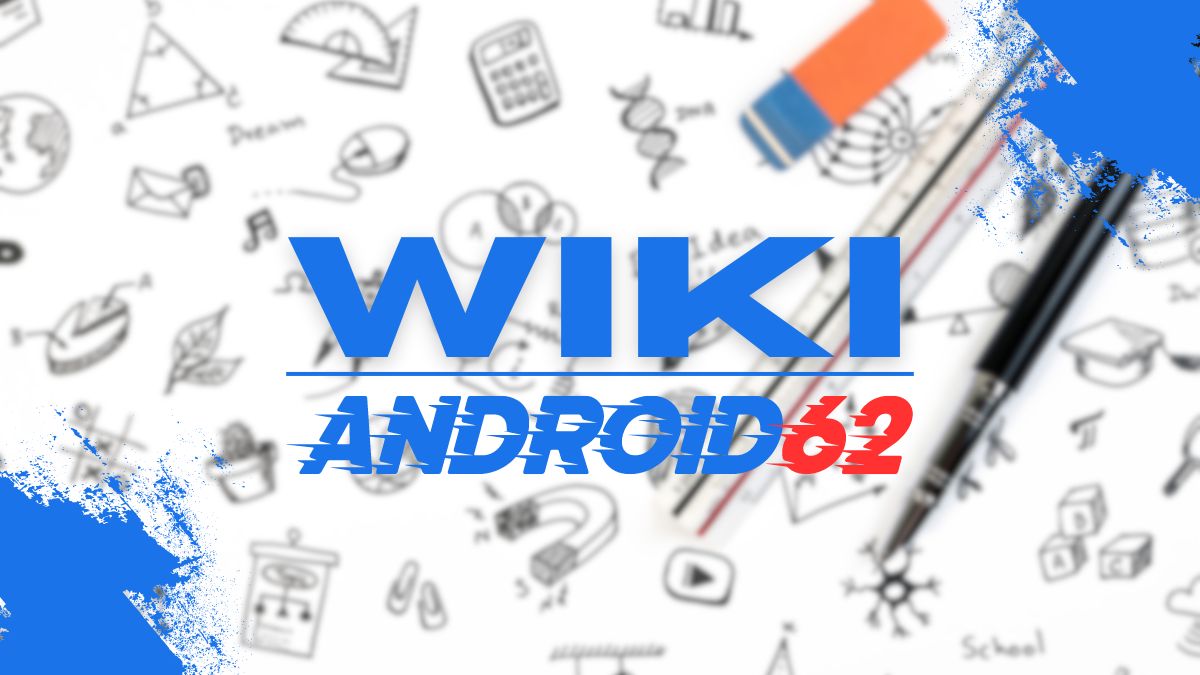
Introduction
When analyzing data points on a graph or scatter plot, determining which line best fits the data is crucial in understanding the relationship and making predictions. In the field of statistics and data analysis, a linear model is often used to represent the relationship between two variables. In this article, we will explore how to identify which line is a linear model for the data and how to interpret the results.
Understanding Linear Models
A linear model is a mathematical representation of the relationship between two variables that can be described by a straight line. It is commonly used in various fields such as economics, physics, and engineering to analyze and predict outcomes based on data points. The equation of a linear model is typically in the form of y = mx + b, where y is the dependent variable, x is the independent variable, m is the slope of the line, and b is the y-intercept.
Identifying the Linear Model
1. Plotting the Data
The first step in identifying which line is a linear model for the data is to plot the data points on a graph or scatter plot. This visual representation helps us understand the relationship between the variables and determine if a linear model is appropriate for the data.
2. Assessing Linearity
To determine if the data follows a linear pattern, visually inspect the scatter plot to see if the points form a straight line. If the data points are roughly aligned in a linear fashion, a linear model may be suitable for the data. However, if the points exhibit a curved or non-linear relationship, a different model may be more appropriate.
3. Calculating the Correlation Coefficient
The correlation coefficient, denoted as r, measures the strength and direction of the linear relationship between two variables. A correlation coefficient close to 1 or -1 indicates a strong linear relationship, while a value close to 0 suggests a weak or no relationship. Calculating the correlation coefficient can help determine if a linear model is suitable for the data.
Interpreting the Results
1. Slope and Intercept
Once the linear model is identified, the slope and intercept of the line can provide valuable insights into the relationship between the variables. The slope m represents the rate of change in the dependent variable for a unit change in the independent variable, while the intercept b indicates the value of the dependent variable when the independent variable is zero.
2. Goodness of Fit
The goodness of fit of a linear model can be assessed using the coefficient of determination, denoted as R2. This value ranges from 0 to 1 and represents the proportion of the variance in the dependent variable that is predictable from the independent variable. A higher R2 value indicates a better fit of the linear model to the data.
3. Residual Analysis
Residual analysis involves examining the differences between the observed values and the predicted values from the linear model. Plotting the residuals against the independent variable can help identify patterns or trends in the data that the model may not capture. A random scatter of residuals around zero indicates that the linear model is appropriate for the data.
Conclusion
In conclusion, determining which line is a linear model for the data involves analyzing the relationship between variables, plotting the data, calculating the correlation coefficient, and interpreting the results. By understanding the characteristics of a linear model and assessing its fit to the data, we can make informed decisions and predictions based on the data points. Remember to consider the slope, intercept, correlation coefficient, coefficient of determination, and residual analysis when identifying the linear model for the data.