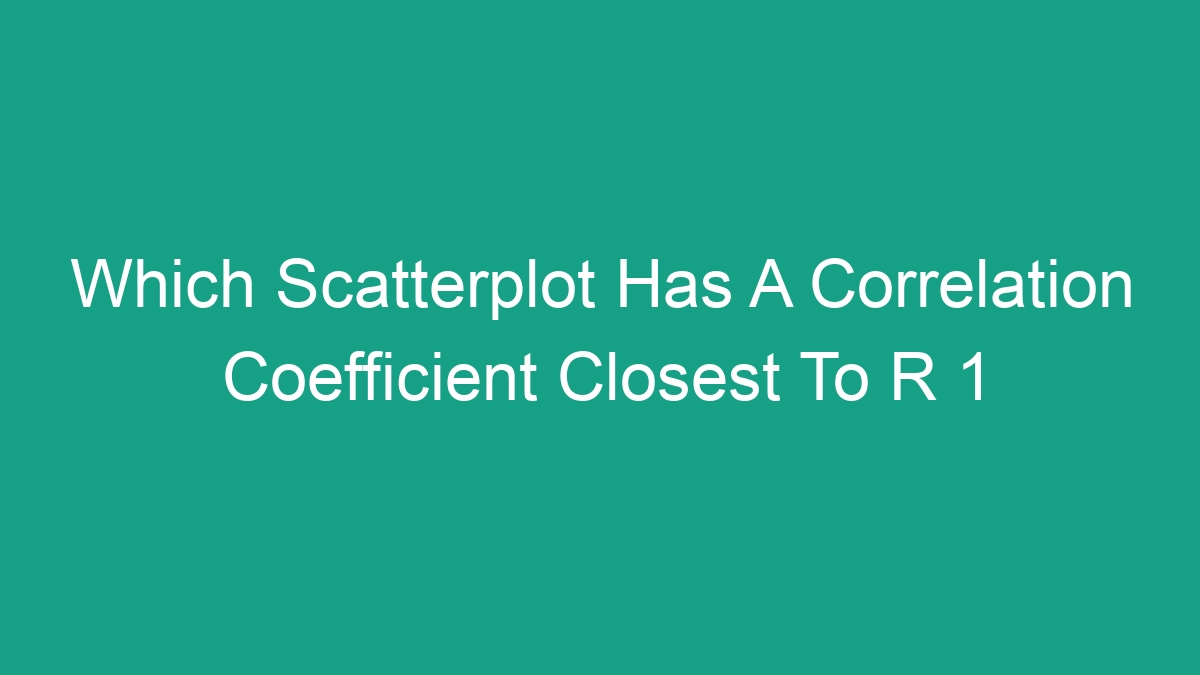
Understanding Correlation Coefficients
Correlation coefficients are used to measure the strength and direction of the relationship between two variables. They range from -1 to 1, with -1 indicating a perfect negative correlation, 0 indicating no correlation, and 1 indicating a perfect positive correlation. When a correlation coefficient is closest to 1, it suggests a strong positive linear relationship between the two variables.
In the context of a scatterplot, a correlation coefficient closest to 1 indicates that the points on the plot tend to fall close to a straight line, with a positive slope. This means that as one variable increases, the other also tends to increase, and vice versa.
Identifying Scatterplots with Correlation Coefficients Closest to 1
When looking at a scatterplot, it can be visually challenging to determine the exact correlation coefficient. However, there are certain characteristics of scatterplots that indicate a strong positive correlation, and therefore, a correlation coefficient closest to 1.
One key characteristic is the tightness and direction of the cluster of points on the plot. If the points form a tightly packed cluster that slants upwards from left to right, it is likely that the correlation coefficient is close to 1. Conversely, if the points form a tightly packed cluster that slants downwards from left to right, the correlation coefficient would be close to -1, indicating a strong negative correlation.
Example of Scatterplot with Correlation Coefficient Closest to 1
Let’s consider an example to illustrate a scatterplot with a correlation coefficient closest to 1. Suppose we have a dataset of students’ study hours and their exam scores. The scatterplot of this data would show study hours on the x-axis and exam scores on the y-axis.
If the scatterplot reveals a tight cluster of points that forms a clear, upward-sloping line, this indicates a strong positive correlation. In this case, the correlation coefficient would be very close to 1, indicating that as study hours increase, exam scores also tend to increase.
Mathematical Calculation of Correlation Coefficients
While visual inspection of a scatterplot can give us a general idea of the correlation coefficient, it is important to understand how to calculate it mathematically.
The most commonly used correlation coefficient is Pearson’s r, which is defined as the covariance of the two variables divided by the product of their standard deviations. The formula for Pearson’s r is:
r = (Σ((X – X̄)(Y – Ȳ))) / (n * σX * σY)
Where:
r = Pearson’s correlation coefficient
X and Y are the two variables
X̄ and Ȳ are the mean of X and Y, respectively
n = number of data points
σX and σY are the standard deviations of X and Y, respectively
Calculating the correlation coefficient using this formula allows for a precise determination of how strong the relationship between the two variables is.
Interpretation of Correlation Coefficients
Once the correlation coefficient is calculated, it is essential to interpret the value to understand the nature of the relationship between the variables. As mentioned earlier, a correlation coefficient closest to 1 indicates a strong positive linear relationship.
If the correlation coefficient is close to 1, it implies that the two variables move in the same direction. This means that as one variable increases, the other variable also tends to increase. On the other hand, if the correlation coefficient is close to -1, it indicates a strong negative linear relationship, where as one variable increases, the other tends to decrease.
Conversely, if the correlation coefficient is close to 0, it suggests that there is no linear relationship between the two variables.
It is crucial to note that correlation does not imply causation. Just because two variables are correlated does not mean that changes in one variable cause changes in the other. There may be confounding variables or other factors at play that need to be taken into consideration.
Practical Applications of Correlation Coefficients
Correlation coefficients have widespread applications in various fields such as economics, psychology, biology, and many others. They are used to measure the strength and direction of relationships between variables, and can provide valuable insights for decision-making and analysis.
In economics, for example, correlation coefficients are utilized to analyze the relationship between variables such as consumer spending and income levels. In psychology, correlation coefficients help researchers understand the connection between behaviors and mental processes. In biology, they are used to study the relationship between environmental factors and biological processes.
Understanding correlation coefficients allows researchers and analysts to make informed conclusions about the relationships between variables, and to identify areas for further investigation and research.
Impact of Outliers on Correlation Coefficients
It is important to note that outliers, or data points that are significantly different from the rest of the data, can have a substantial impact on correlation coefficients. Outliers can skew the results and give a false impression of the strength of the relationship between the variables.
For example, if a scatterplot shows a strong positive correlation, but there is one outlier that is significantly lower than the rest of the data points, it can substantially reduce the correlation coefficient. In such cases, it is vital to investigate the cause of the outlier and consider whether it should be included in the analysis.
Conclusion
In conclusion, identifying a scatterplot with a correlation coefficient closest to 1 requires an understanding of the visual characteristics of the plot, as well as the mathematical calculation and interpretation of correlation coefficients. Correlation coefficients provide valuable insights into the relationships between variables, and can be used in a wide range of fields to make informed decisions and draw meaningful conclusions.
When analyzing a scatterplot, pay attention to the tightness and direction of the points, and consider the mathematical calculation of the correlation coefficient to determine the strength of the relationship between the variables. Remember to interpret the correlation coefficient in the context of the specific variables being studied, and consider the potential impact of outliers on the results.
By understanding and utilizing correlation coefficients effectively, researchers, analysts, and decision-makers can gain valuable insights and make informed decisions based on the relationships between variables.