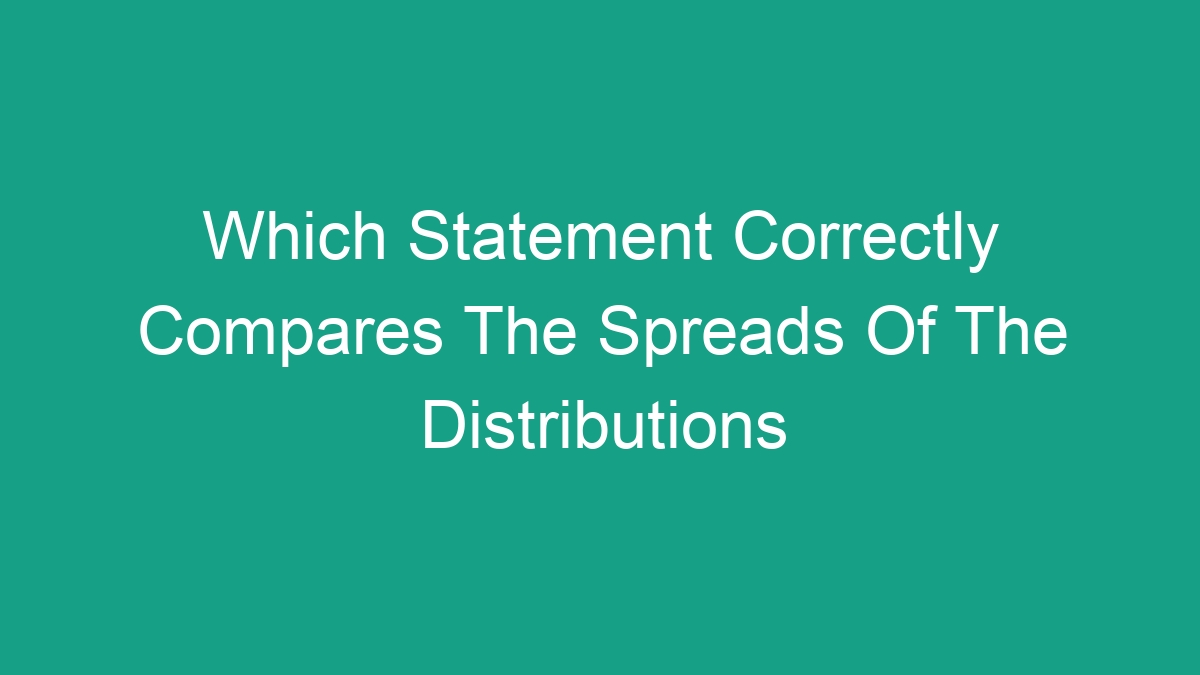
Understanding Distribution Spreads
When it comes to statistics and data analysis, the spread of a distribution is an essential concept. The spread refers to the extent to which the values in a dataset differ from the mean or median. A wider spread indicates that the values are more dispersed, while a narrower spread indicates that the values are more clustered around the central measure.
There are several measures that can be used to quantify the spread of a distribution, including the range, variance, and standard deviation. Understanding these measures and knowing how to compare the spreads of different distributions is crucial for making informed decisions in various fields, including finance, economics, and quality control.
The Range as a Measure of Spread
One of the simplest measures of spread is the range, which is the difference between the largest and smallest values in a dataset. While the range provides a straightforward way to assess the spread, it can be heavily influenced by outliers, which are extreme values that lie far from the rest of the data. As a result, the range may not always provide an accurate representation of the overall spread of the distribution.
It’s important to note that the range only considers two data points, the maximum and minimum, and does not take into account the distribution of values in between. For a more comprehensive understanding of the spread, it’s often necessary to consider other measures such as the variance and standard deviation.
Variance and Standard Deviation
Variance and standard deviation are two widely used measures of spread that provide a more nuanced assessment of the distribution. Variance is calculated by taking the average of the squared differences between each data point and the mean, while the standard deviation is the square root of the variance. These measures provide a more precise indication of how the values are dispersed around the central measure.
It’s important to note that the standard deviation is often preferred over the variance because it is expressed in the same units as the original data, making it easier to interpret and compare.
Using these measures, it becomes possible to compare the spreads of different distributions with greater accuracy. However, it’s crucial to ensure that the distributions being compared are of the same type and are based on similar data. In addition, understanding the shape of the distributions is also important, as distributions with the same spread may still have different characteristics.
Comparing the Spreads of Distributions
When comparing the spreads of two distributions, several statements can be used to accurately determine how the spreads differ. The correct statement will depend on the specific characteristics of the distributions being compared, as well as the measures used to quantify the spread.
1. If the standard deviation of distribution A is larger than the standard deviation of distribution B, it can be said that the values in distribution A are more spread out than the values in distribution B.
2. If the ranges of distribution A and distribution B are equal, but the variance of distribution A is larger than the variance of distribution B, it can be said that the values in distribution A are more dispersed than the values in distribution B.
3. If the ranges of distribution A and distribution B are equal, and the variances of distribution A and B are also equal, but the standard deviation of distribution A is larger than the standard deviation of distribution B, it can once again be said that the values in distribution A are more spread out than the values in distribution B.
It’s important to note that when comparing the spreads of distributions, it’s crucial to consider the context in which the data is being used. A larger spread may not always be undesirable, and in some cases, it may be indicative of greater variability, which could be valuable information.
Real-World Applications
Understanding how to accurately compare the spreads of distributions is essential in various real-world applications. In finance, for example, comparing the spreads of stock returns can provide valuable insights into the volatility and risk associated with different investments. A wider spread may indicate a higher degree of risk, while a narrower spread may suggest more stability.
In manufacturing and quality control, comparing the spreads of product measurements can help identify potential issues with consistency and uniformity. A wider spread may indicate greater variability in the production process, which could lead to quality control problems.
In healthcare, comparing the spreads of patient outcomes can provide important information about the effectiveness of different treatments and interventions. A wider spread may indicate a wider range of responses to a particular treatment, which could inform future healthcare decisions.
Conclusion
Understanding the spread of a distribution and knowing how to compare the spreads of different distributions is crucial for making informed decisions in various fields. By utilizing measures such as the range, variance, and standard deviation, it becomes possible to accurately assess the extent to which values in a dataset differ from the central measure.
When comparing the spreads of distributions, it’s important to consider the specific characteristics of the data, as well as the context in which the comparisons are being made. By doing so, it becomes possible to draw meaningful conclusions and make informed decisions based on the spread of the data.
Ultimately, understanding how to compare the spreads of distributions provides valuable insights that can be applied to a wide range of real-world applications, from finance and economics to healthcare and quality control.